Artificial Intelligence (AI) is transforming the world, but as it becomes more embedded in our daily lives, it also introduces significant risks to data security.
AI-powered systems, from personal assistants to automated decision-making tools, are revolutionizing industries by offering enhanced efficiency, predictive capabilities, and problem-solving.
However, they also expose new vulnerabilities. The dangers of AI security risks—such as AI-powered hacking and adversarial machine learning attacks—are growing faster than the tools to protect against them.
As businesses and individuals rely more on AI, it’s crucial to understand how AI security risks can affect data protection and what steps can be taken to mitigate them.
In this blog, we will explore the increasing number of AI-driven cyber threats, their impact on various industries, and, most importantly, how to protect your systems from AI vulnerabilities.
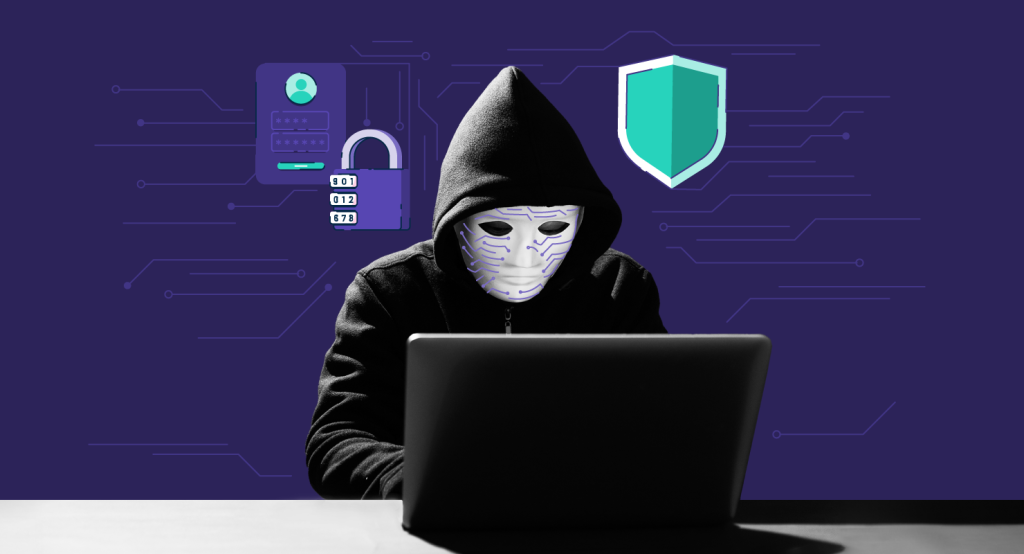
What Are AI Security Risks?
AI security risks refer to the vulnerabilities inherent in AI systems that can be exploited by cybercriminals or attackers. These risks are unique because AI systems learn and evolve over time, which makes them prone to errors and manipulation.
Common examples include AI-powered hacking risks, adversarial attacks on machine learning models, and vulnerabilities in AI-based systems for data protection.
AI systems depend on vast amounts of data to function correctly. Unfortunately, this makes them an attractive target for cybercriminals who can exploit AI vulnerabilities to gain unauthorized access to sensitive data.
From automated malware attacks to AI-driven phishing scams, the rise of AI in cybersecurity is both a blessing and a curse.
The Dual Nature of AI: Opportunities and Threats
AI has the potential to revolutionize cybersecurity by automating threat detection, enabling predictive capabilities, and improving incident response. However, these advancements also introduce significant risks.
Cybercriminals can weaponize AI, resulting in AI-driven cyber threats that traditional security measures are ill-equipped to handle. The risks of AI in cybersecurity are escalating as more sophisticated and diverse AI-powered hacking risks emerge.
The dual nature of AI is best understood by recognizing that the same technology that improves security can also introduce weaknesses.
For example, while AI can detect security breaches quickly, attackers can use AI to identify and exploit flaws in security systems. The key challenge here is to secure AI systems from attacks while using them to improve security.
LEARN MORE ABOUT REBOOT MONKEY
Why Are AI Risks Growing Rapidly?
The growth of AI risks is not just about the technology itself—it’s also about the scale at which AI is being implemented.
As AI becomes more integrated into our systems, industries, and daily lives, the scale of potential damage increases.
Below are several key reasons why AI security risks are growing rapidly.
The Rise of Edge Computing: Driving Low-Latency Services with IoT and 5G | Reboot Monkey
- Increasing Complexity of AI Systems
As AI systems evolve, they become more complex, learning from vast datasets and adapting their behavior over time. This increasing complexity makes it harder to predict and prevent security vulnerabilities. Attackers are quick to find these weaknesses and exploit them for malicious purposes.
- Expanded Use of AI Across Industries
The widespread use of AI across industries, including healthcare, finance, manufacturing, and government, increases the attack surface for cybercriminals.
The more AI is used the more points of entry for potential cyber threats. Every sector faces unique AI security risks, from AI-powered financial fraud detection systems to healthcare patient records.
- Lack of Comprehensive Regulation
While AI technology is evolving rapidly, regulations governing AI use and security have not kept pace. Many organizations are left to their own devices when securing AI systems, leaving significant gaps in cybersecurity protocols. This lack of regulation increases the likelihood of AI vulnerabilities being exploited.
- High Value of AI Data
AI systems rely on massive datasets, often containing sensitive information like personal details, financial data, or trade secrets.
The value of this data makes AI systems an attractive target for cybercriminals looking to steal valuable information. AI-powered hacking risks are growing as attackers increasingly target these valuable datasets.
Industry-Specific AI Security Risks
Different industries are encountering unique AI security risks due to the specific nature of the data and systems they use. Here’s a closer look at how AI security risks impact various sectors:
Financial Sector: AI and Fraud Detection Loopholes
The financial sector relies heavily on AI for fraud detection and risk management. AI algorithms analyze transaction patterns to detect anomalies and flag potential fraud. However, as AI systems become more advanced, so do fraudsters’ tactics.
AI-driven cyber threats are increasingly being used to bypass these AI-powered fraud detection systems. Attackers can use machine learning techniques to “train” AI models to recognize and avoid detection, making it harder for banks to identify fraud in real-time.
Additionally, machine learning security issues may arise when banks fail to properly secure the models used for fraud detection, leaving them vulnerable to manipulation. These vulnerabilities create loopholes that cybercriminals can exploit to carry out financial fraud.
Healthcare: Protecting Patient Data from AI Exploits
In the healthcare industry, AI enhances diagnostics, predicts patient outcomes, and manages medical records. However, the vast amount of sensitive patient data used by AI systems makes them a prime target for AI-powered hacking risks.
Hackers who gain access to an AI system can manipulate the data to alter medical records, compromise patient privacy, or cause misdiagnoses.
AI vulnerabilities in data protection are particularly concerning in healthcare, as the stakes are extremely high. Ensuring that AI systems are secure and that patient data remains protected from exploits is a top priority for the healthcare sector.
Manufacturing: Securing Smart Systems
Manufacturing industries are adopting AI-powered automation to improve productivity and streamline operations. However, the increasing reliance on smart systems and AI-powered machinery introduces new security risks.
Machine learning security issues can occur when these systems are hacked or manipulated, potentially leading to production downtime, quality control issues, or physical safety hazards.
AI vulnerabilities can also extend to the supply chain, as automated systems manage everything from inventory to logistics. Securing these AI-driven systems from attacks is crucial for maintaining operational integrity.
Government: National Security Challenges
Governments use AI for various national security purposes, from monitoring surveillance data to managing defense systems. However, these systems are highly susceptible to adversarial AI attacks.
AI vulnerabilities in data protection could lead to attacks on critical infrastructure, intelligence operations, or even national elections.
The risks of AI in cybersecurity are significant in the government sector, as malicious actors may seek to manipulate AI systems to compromise national security. Protecting AI-powered government systems from hacking is a matter of national importance.
Common AI-Driven Cyber Threats
AI technology has introduced innovative ways to combat cybercrime, but it has also empowered attackers with new tools to exploit vulnerabilities. Below are some of the most pressing AI-driven cyber threats that individuals and organizations must be aware of:
AI-Powered Phishing and Social Engineering
Traditional phishing attacks rely on generic emails and fake websites to trick users into revealing sensitive information. However, AI-powered phishing campaigns take these attacks to the next level. AI can use advanced data analysis to create highly personalized phishing emails tailored to the victim’s behavior, interests, and communication style.
Example:
For example, an AI-driven attack might analyze your social media activity to craft an email referencing a recent event in your life, making the message seem genuine.
These sophisticated phishing scams are harder to detect because they mimic legitimate correspondence’s tone, context, and language.
Social engineering attacks driven by AI can extend to impersonating high-ranking officials or colleagues, putting corporate data and personal privacy at significant risk.
Adversarial Machine Learning Attacks
Adversarial machine learning is a technique in which attackers subtly manipulate an AI model’s input data to deceive it into making incorrect decisions. These attacks exploit the way AI models process and interpret data, leading to potentially catastrophic consequences.
Example:
For instance, consider an AI-powered facial recognition system used in security. An attacker could trick the system into misidentifying an individual by altering a small portion of the input image—such as adding a few inconspicuous patterns.
Similarly, adversarial attacks on AI models in healthcare could lead to misdiagnoses or improper treatment recommendations. The potential for misuse in adversarial machine learning poses significant risks for industries relying on AI.
Deepfake Technology and Its Implications
Deepfake technology, fueled by AI, allows attackers to create highly realistic fake videos or audio recordings that are nearly indistinguishable from authentic ones. These manipulated media can have far-reaching consequences, from political misinformation campaigns to corporate espionage.
Example:
Imagine a scenario where a deepfake video of a company CEO announces false financial information, leading to a stock market crash.
Alternatively, cybercriminals might use deepfake audio to impersonate executives and authorize fraudulent transactions—a phenomenon already known as “CEO fraud.”
The potential misuse of deepfake technology raises serious ethical concerns in AI security as it becomes increasingly difficult to trust what we see and hear.
Automated Malware and Ransomware
AI enables the automation of malware and ransomware creation, allowing attackers to produce malicious software at an unprecedented scale.
Unlike traditional malware, AI-powered malware can learn and adapt to evade detection by antivirus systems. These programs analyze security protocols in real-time, modifying their behavior to bypass defenses.
Example:
For example, thanks to AI, ransomware attacks, which encrypt a victim’s data until a ransom is paid, have become more sophisticated.
Automated ransomware campaigns can target multiple organizations simultaneously, dynamically adapting their strategies based on the victim’s response. The combination of AI and automation makes these threats more dangerous and harder to contain.
AI-Powered Botnets
Botnets are networks of compromised devices controlled remotely by attackers, often used for distributed denial-of-service (DDoS) attacks. With the integration of AI, these botnets have become more intelligent and efficient.
AI-powered botnets can identify and exploit vulnerabilities faster, adapt their attack patterns to avoid detection and launch highly coordinated attacks against multiple targets.
Example:
For instance, an AI-driven botnet might monitor the target’s network traffic to determine the optimal time to strike, ensuring maximum disruption.
These advanced botnets are a significant threat to organizations, as traditional cybersecurity measures often struggle to maintain their adaptive capabilities.
AI in Identity Theft and Fraud
Identity theft has been a longstanding issue in cybersecurity, but AI has added a new layer of complexity. Cybercriminals now use AI to gather personal information from public and private sources, creating detailed profiles of their targets.
With this information, attackers can impersonate individuals with alarming accuracy, committing fraud or gaining unauthorized access to sensitive systems.
Example:
For example, AI can generate convincing fake identities that pass background checks or fool biometric security systems. Additionally, attackers can use stolen identities to open fraudulent accounts, apply for loans, or even commit crimes in someone else’s name.
The impact of AI-powered identity theft extends beyond financial losses, damaging reputations and creating long-lasting legal issues for victims.
AI in Cyber Espionage
State-sponsored attackers and cybercriminal organizations are increasingly using AI for cyber espionage. These sophisticated attacks leverage AI to infiltrate networks, gather intelligence, and exfiltrate sensitive data without detection.
AI-powered espionage tools can analyze vast amounts of data to identify valuable information and locate vulnerabilities in a target’s systems.
Example:
One prominent example is the use of AI to monitor communications and detect keywords or patterns that indicate high-value intelligence.
These capabilities allow attackers to focus their efforts on specific targets, increasing cyber espionage campaigns’ efficiency and success rate.
AI and Machine Learning in Data Centers: Transforming Efficiency and Security | Reboot Monkey
Predictive Cyberattacks
AI’s ability to predict future trends isn’t limited to legitimate applications. Cybercriminals are using AI to anticipate and exploit potential vulnerabilities in cybersecurity systems.
AI-powered tools can predict where and when new vulnerabilities will arise by analyzing patterns in security updates, patch releases, and network behavior.
Example:
For instance, if a company frequently delays software updates, an AI-driven attack might target those delays to exploit unpatched vulnerabilities. Predictive cyberattacks are proactive and highly efficient, making them a significant challenge for cybersecurity teams.
Mitigating AI Risks in Data Security:
As AI security risks continue to evolve, implementing effective mitigation strategies is crucial for protecting sensitive data and ensuring the ethical use of AI systems.
Here are several measures organizations can adopt to address AI vulnerabilities in data protection and safeguard against AI-driven cyber threats.
Building Resilient AI Systems
The foundation of mitigating AI risks lies in designing resilient systems capable of withstanding sophisticated attacks. This involves integrating robust security measures into every stage of the AI development lifecycle, from data collection to deployment. Resilient systems must:
- Enhance Data Security Protocols:
Ensure that all data used to train AI models is encrypted and anonymized. This reduces the risk of exposure in case of a breach. Additionally, organizations should implement access controls to limit who can interact with sensitive datasets.
- Conduct Adversarial Testing:
To identify vulnerabilities, regularly test AI systems against adversarial machine learning attacks. Simulating such attacks can help developers understand how AI models respond to manipulated inputs and reinforce defenses.
- Implement AI-Specific Firewalls:
Traditional cybersecurity measures often fail to address machine learning security issues. AI-specific firewalls, which monitor and filter anomalous activity, can act as an additional layer of protection against AI vulnerabilities in data protection.
How AI at the Edge is Revolutionizing Real-Time Decision Making | Reboot Monkey
Regulatory and Ethical Safeguards
Addressing the ethical concerns in AI security risks is as important as tackling technical challenges. Ethical considerations ensure that AI systems are used responsibly and transparently, fostering trust among users and stakeholders. Governments and regulatory bodies play a key role in this process.
- Compliance with Global Standards:
Organizations should align their AI systems with industry standards such as GDPR (General Data Protection Regulation) or CCPA (California Consumer Privacy Act) to ensure compliance with data privacy laws. Following these regulations not only safeguards data privacy but also minimizes the risks of AI in cybersecurity misuse.
- Ethical AI Frameworks:
Companies should adopt frameworks that prioritize accountability, fairness, and transparency in AI development. Guidelines that discourage bias and prevent the misuse of AI in decision-making processes can mitigate ethical concerns about AI security.
Employing AI to Combat AI-Driven Threats
One of the most promising approaches to mitigating AI-powered hacking risks is to leverage AI itself. Organizations can harness AI’s predictive capabilities to detect and neutralize threats before they materialize.
- AI-Powered Threat Detection:
AI systems can monitor network traffic and user behavior in real time, identifying anomalies that could indicate an attack. By analyzing large datasets, AI can recognize patterns associated with AI-driven cyber threats, such as phishing attempts or automated malware.
- Self-Healing Systems:
Developing self-healing systems can address Machine learning security issues. These AI-driven systems can identify vulnerabilities, patch them automatically, and adapt to evolving threats without human intervention. This reduces the window of opportunity for attackers and ensures continuous protection.
Continuous Security Audits
Routine security audits are vital for keeping AI systems secure. These audits involve assessing the organization’s cybersecurity infrastructure to identify weaknesses and areas for improvement. Key steps include:
- Penetration Testing:
Ethical hackers can simulate AI-powered hacking risks to expose vulnerabilities in a controlled environment. This helps organizations understand their weak points and implement the necessary safeguards.
- Model Updates and Monitoring:
AI vulnerabilities in data protection often arise due to outdated models. Regularly updating AI systems ensures they stay ahead of emerging threats. Additionally, continuous monitoring allows organizations to detect and address anomalies in real time.
- Third-Party Audits:
Independent audits by cybersecurity experts can provide an unbiased assessment of an organization’s AI security risks measures. This helps identify gaps that internal teams might overlook and provides actionable recommendations.
Promoting Awareness and Training
Addressing the risks of AI in cybersecurity is not just a technical challenge—it’s also a human one. Employees and stakeholders must understand the potential dangers of AI vulnerabilities and their role in preventing them.
- Employee Training Programs:
Regular training sessions can educate employees about common AI-driven cyber threats like phishing and social engineering attacks. Awareness empowers individuals to recognize and report suspicious activities before they escalate.
- AI Ethics Workshops:
To tackle ethical concerns in AI security, organizations can host workshops that discuss responsible AI usage. These sessions can foster a culture of accountability and ensure that ethical principles guide decision-making processes.
Collaboration Across Industries
No single organization can combat AI vulnerabilities in data protection alone. Cross-industry collaboration is essential for sharing knowledge, resources, and best practices.
- Information Sharing Platforms:
Industries can create platforms to share insights on emerging AI-driven cyber threats. Organizations can stay informed about the latest attack trends and mitigation strategies by pooling data.
- Public-Private Partnerships:
Governments and private companies can collaborate to develop policies and technologies that address AI’s impact on data privacy. These efforts can lead to innovative solutions that balance innovation and security.
Investing in Advanced Technologies
To effectively counter AI vulnerabilities, organizations must invest in advanced security technologies. Emerging tools such as quantum encryption and AI-driven risk assessment platforms can provide additional layers of protection.
- Quantum Encryption:
While still in its early stages, quantum encryption offers unparalleled security, making it nearly impossible for attackers to intercept data. Organizations exploring cutting-edge solutions can stay ahead of the curve in mitigating AI security risks.
- AI Risk Assessment Tools:
These tools can analyze an organization’s cybersecurity posture, identifying potential risks and recommending specific actions to address them. Organizations can proactively mitigate machine learning security issues by integrating these tools into their operations.
Conclusion
AI security risks are real and escalating. As organizations and individuals increasingly rely on AI, it’s crucial to understand the vulnerabilities these technologies introduce. Whether you’re securing personal data, financial transactions, or critical infrastructure, protecting against AI-driven cyber threats is a top priority.
At Reboot Monkey, we specialize in safeguarding your systems against the growing risks of AI vulnerabilities. Our AI-driven solutions offer the protection you need to stay ahead of emerging threats.
Contact Reboot Monkey today to learn more about securing your AI systems and ensuring your data stays safe.
FAQs:
1. What are the main AI security risks businesses face today?
AI security risks include adversarial machine learning attacks, data poisoning, AI-powered phishing, deepfake technology, and automated malware.
2. How do AI-driven cyber threats differ from traditional cybersecurity risks?
AI-driven cyber threats are more sophisticated and adaptive compared to traditional risks. Attackers use AI to analyze vulnerabilities, create targeted phishing campaigns, and automate malware attacks.
3. What steps can organizations take to mitigate AI vulnerabilities in data protection?
Organizations should implement resilient AI systems to mitigate AI vulnerabilities, conduct continuous security audits, and adhere to ethical and regulatory safeguards.
4. How does deepfake technology impact data privacy and security?
Deepfake technology creates realistic fake videos and audio, which can be used for identity theft, spreading misinformation, or committing fraud.
5. What role does AI play in shaping the future of cybersecurity?
AI is both a risk and a solution in cybersecurity. While it introduces new vulnerabilities, it also enhances threat detection and response capabilities.
LEARN MORE ABOUT US